Summer Seminar Speaker
Join our mailing list to receive the Seminar Zoom links via email.
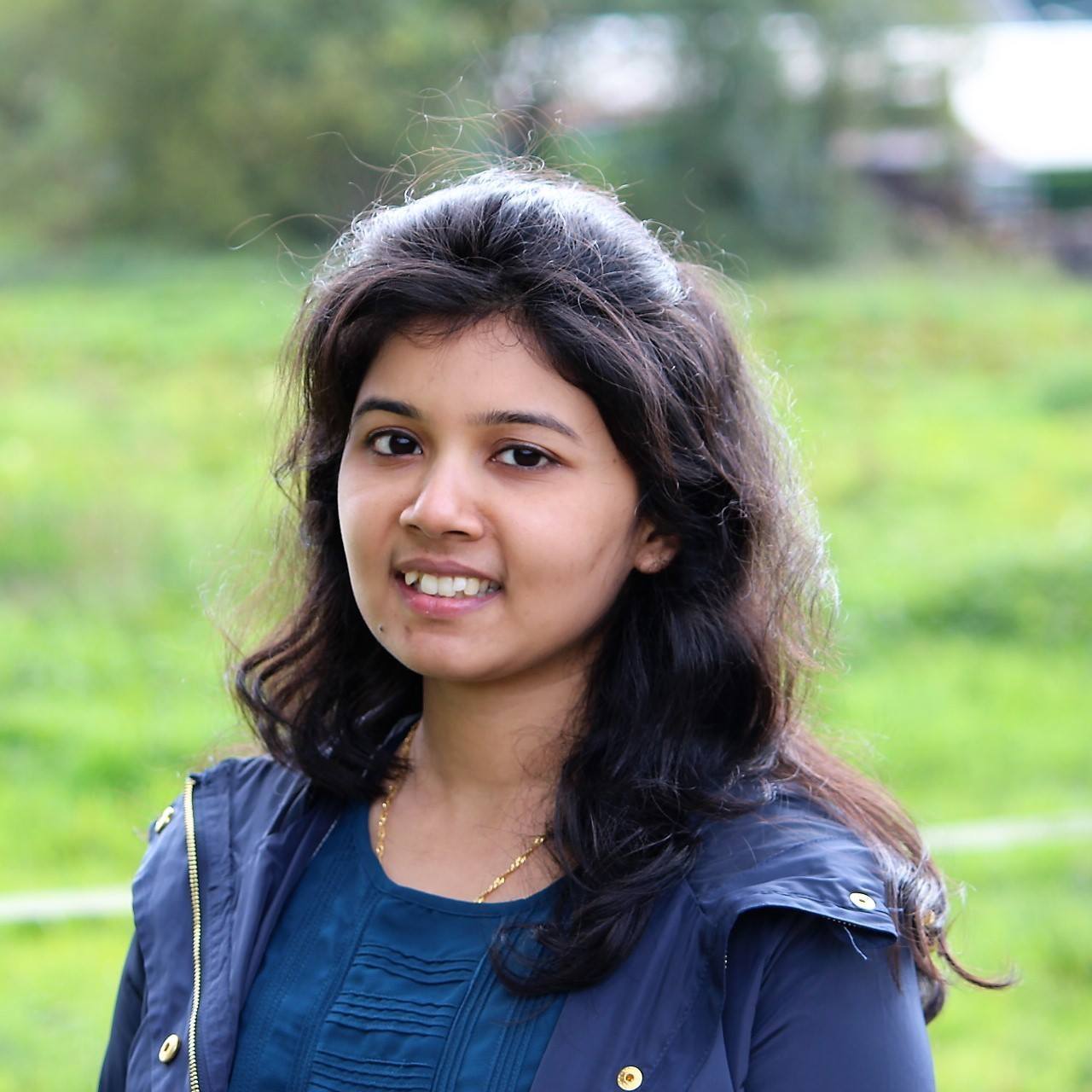
Arpita Biswas
Presenting June 12, 2020 @ 11:30 am MDT
Poster:
See PDF here
Talk Title: Two-Sided Fairness Guarantees for Recommendation Systems (arXiv)
Abstract:
Major B2C eCommerce websites (such as Amazon, Spotify, Swiggy) can be thought of as two-sided platforms, with customers on one side and producers on the other. Traditionally, recommendation protocols of these platforms are customer-centric---focusing on maximizing customer satisfaction by tailoring the recommendation according to the personalized preferences of individual customers. However, this may lead to unfair distribution of exposure among the producers and adversely impact their well-being. As more and more people depend on such platforms to earn a living, it is important to strike a balance between fairness among the producers and customer satisfaction. The problem of two-sided fairness in recommendation can be formulated as a matroid constrained fair allocation problem. This problem naturally captures a number of other resource-allocation applications, including budgeted course allocation, graph partition, and allocation of cloud computing resources. Our main contribution is to develop polynomial time algorithms for fair allocation of indivisible items (with formal guarantees on fairness and feasibility) in several practical scenarios which can be formulated as matroid constrained problems.
In this talk, I’ll start by motivating the problem and then defining some popular fairness notions. Next, I’ll discuss the matroid constrained fair allocation problem, and show how the solutions can be applied to ensure two-sided fair recommendation. I’ll conclude by listing down some open problems.
Bio:
Arpita Biswas is a Google Ph.D. Fellow at the Department of Computer Science and Automation, Indian Institute of Science. Her broad areas of interest include Game Theory, Optimization, and Machine Learning. She is presently looking at problems in Computational Social Choice Theory and Fairness in Machine Learning. Prior to her Ph.D., she worked as a Research Engineer at Xerox Research Centre India. Her work spans several research spaces such as multi-agent learning, incentive mechanisms, facility location, planning and scheduling, etc. Thus far, she has worked on problems arising from real-world scenarios like online crowd-sourcing, resource allocation, dynamic pricing in transportation, and ride-sharing. More details about her can be obtained at https://sites.google.com/view/arpitabiswas/.